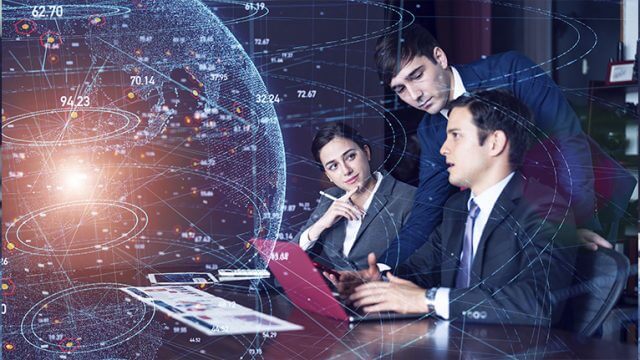
E-Invoicing and Its Geopolitical Stakes
France’s electronic invoicing reform relies on a Y-architecture, where Partner Dematerialization Providers (PDPs) play a central role in issuing and…
Medial International Chooses Generix Warehouse Management System (WMS) to Digitalize Its Logistics Operations Read the press release
Ayoub Mcharek, Data Lab Manager for Generix Group, provides this preliminary assessment of the use of data and artificial intelligence in the supply chain:
“AI offered new solutions to well-known challenges, especially for demand forecasting, a key element of efficiency across the whole supply chain. Although multiple algorithms are available to help forecast demand levels, especially in the mass retail and fashion industries, it remains an extremely complex practice which involves many factors. In this regard, machine learning has enabled forecast quality to make great leaps forward.”
Our expert points out that AI has also provided answers to problems that had remained unsolved until now, such as computer vision to assess stock levels via surveillance camera images. “We can imagine there will come a day when algorithms will track every item movement in the warehouse via a camera system, as in Amazon’s cashier-less stores,” adds Ayoub Mcharek.
While AI has in some way become the standard-bearer for data use in business, there are already many optimization algorithms at each logistical step. Gartner broadly describes four stages of data maturity. The first, analytics and its popular dashboards, are already well-known and commonplace in supply chain management. The data supplied in this case is purely descriptive: its purpose is to show decision-makers the key figures representing their supply chain activity. Algorithms complement this approach by revealing trends and explaining the numbers. However, since people perform most of the analysis, the role of the algorithm is to deliver a diagnostic.
Thanks to predictive analytics, AI leverages past data to show expected short and long-term changes. Although this information is especially relevant for decision-making, a person is still responsible for making that decision. Prescriptive systems are the ultimate stage of AI’s evolution in supply chain management. Those algorithms will make recommendations to optimize operations. These recommendations will further enhance the decision-making process, or they will simply become part of the automation. The algorithms will make the decisions to manage the supply chain’s day-to-day operations, while human intervention will only be needed to deal with exceptions.
“The implementation of AI requires as much data about the supply chain as possible,” emphasizes Ayoub Mcharek. “This is why we recommend retaining historical data. This historical information will allow us to train models capable of predicting the future, extrapolating values, and identifying and classifying information.” Logistics data recorded and retained during the COVID crisis or since the beginning of the war in Ukraine will make it possible to improve our predictions of the impact of a future pandemic or some other global event on the supply chain.
By storing all its customers’ anonymized data in the same data lake, Generix has access to as much information as possible to offer companies the most efficient algorithms. “The data aggregated from three to four thousand warehouses belonging to several hundred companies with a wide variety of profiles allows us to train our models with a much greater data volume than could ever be collected by a single company.” In the future, access to this massive amount of anonymized data will help benchmark a warehouse’s efficiency against similar facilities belonging to other companies in the same industry. Mcharek envisions: “In the future, this data will help determine which processes are to be integrated into a warehouse, for example via its WMS, thereby using AI recommendations to accelerate the tool’s integration.”
To develop new supply chain use cases, it is critical to store data collected from multiple logistical components involved in the process. “By correlating data supplied by the software and services involved at every step of the supply chain, we can reach a new level of optimization,” notes Ayoub Mcharek. “For example, we can merge YMS and WMS data to optimize truck assignments at the loading and unloading docks”.
Or in the case of TMS, several providers offer algorithms to estimate delivery arrival times, the so-called ETA (Estimated Time of Arrival).“ETA is a very useful key indicator for transport management and end consumers. From a competitive standpoint, e-merchants that provide better visibility and more accurate delivery dates will stand a better chance of winning the deal. Until now, the most efficient algorithms and systems available on the market are not delivering accurate upstream results, except for a few players like Amazon. An overwhelming number of European companies cannot provide customers with this level of visibility. It requires factoring all current data and processes, such as shipment preparation time, real-time availability of the warehouse loading dock, loading time, planned and ongoing delivery routes, etc. A digital system that connects players in this increasingly complex logistics ecosystem, such as the solution suite offered by Generix (WMS, TMS, YMS, SCV, GCR, AIO), can help businesses meet this need more effectively. The data and information collected in our various seamlessly integrated modules can power our algorithms and deliver a much more accurate ETA”.
Leveraging AI to collect data throughout the supply chain, understanding this data, identifying correlations and trends, extracting useful information for customers and helping them make pragmatic decisions are this decade’s digital challenges and must become a priority in 2023.
Artificial intelligence has shown that it can offer several new solutions to optimize processes, and Generix offers machine learning and deep learning algorithms to all its customers. These functionalities are ready to help them take a step forward in supply chain optimization and carbon footprint reduction. However, there is still much to explore, notes Ayoub Mcharek enthusiastically: “We are already working on the next disruptive technology for supply chain optimization: quantum computing. We are setting up a quantum pilot with a specialized start-up, Qbitsoft. Our goal is to provide new logistics solutions in the current ecological context. This new paradigm could enable another leap forward in complex calculation processing speed. We hope to significantly reduce the carbon footprints of logistics companies in the future”.
France’s electronic invoicing reform relies on a Y-architecture, where Partner Dematerialization Providers (PDPs) play a central role in issuing and…
The B2B mandate in Germany, set to take effect on January 1, 2025, marks a crucial step in the European…
Following the October 15 announcement regarding the abandonment of the PPF development, the DGFIP and its partner AIFE are ramping…
Work with our team to build your ideal supply chain software stack and tailor it to your unique business needs.